WaveNet by Google DeepMind | Two Minute Papers #93 | Summary and Q&A
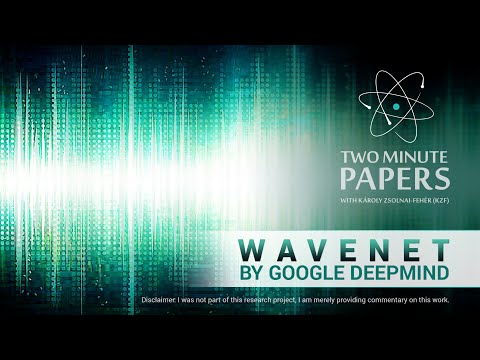
TL;DR
WaveNet is a novel approach to generating audio waveforms for Text to Speech using dilated convolutions in a convolutional neural network.
Key Insights
- 😒 WaveNet uses dilated convolutions in a convolutional neural network to generate audio waveforms for Text to Speech, resulting in more accurate and human-like speech synthesis.
- 😯 The technique outperforms existing concatenative synthesis methods in terms of generating more natural and consistent speech outputs.
- 👂 WaveNet has the potential for various applications beyond Text to Speech, including music generation and artistic style transfer for sound and instruments.
- 🚂 Training a convolutional neural network for audio synthesis is easier and more efficient than training a recurrent neural network.
- ✊ WaveNet demonstrates the power of deep learning in tackling challenging problems in audio processing.
- 👂 The algorithm currently takes 90 minutes to synthesize one second of sound waveforms, but future advancements are expected to improve its efficiency.
- 🤗 The results of WaveNet open up possibilities for more advanced and realistic voice synthesis techniques in the future.
Transcript
Dear Fellow Scholars, this is Two Minute Papers with Károly Zsolnai-Fehér. When I opened my inbox today, I was greeted by a huge deluge of messages about WaveNet. Well, first, it's great to see that so many people are excited about these inventions, and second, may all your wishes come true as quickly as this one! So here we go. This piece of work ... Read More
Questions & Answers
Q: How does WaveNet differ from traditional Text to Speech techniques?
WaveNet differs from traditional techniques by using dilated convolutions instead of recurrent neural networks, allowing for sample-by-sample generation of audio waveforms. This results in more accurate and human-like speech synthesis.
Q: How does WaveNet achieve better global understanding of the input data?
WaveNet achieves better global understanding of the input data by utilizing dilated convolutions, which allow for large skips in the input data. This increases the receptive field of the model, similar to increasing the field of view of the human eye in computer vision.
Q: What are the limitations of existing techniques like concatenative synthesis?
Existing techniques like concatenative synthesis have limitations in generating natural and human-like speech outputs. They often sound robotic and lack the flexibility of producing non-speech sounds like breathing and mouth movements.
Q: What are the potential applications of WaveNet beyond Text to Speech?
WaveNet has potential applications in music generation and artistic style transfer for sound and instruments. It could also be used for creating audiobooks automatically, as well as other voice synthesis applications.
Summary & Key Takeaways
-
WaveNet is a technique for generating audio waveforms for Text to Speech, allowing for voice synthesis in someone's voice if training samples are available.
-
The technique uses dilated convolutions in a convolutional neural network to generate waveforms sample by sample at a high rate of 16 or 24 thousand samples per second.
-
It outperforms existing techniques, such as concatenative synthesis, in terms of generating more human-like and consistent outputs.
Share This Summary 📚
Explore More Summaries from Two Minute Papers 📚
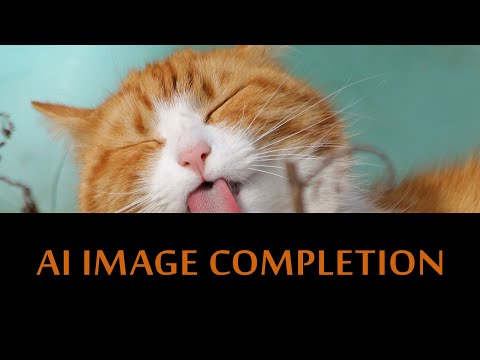
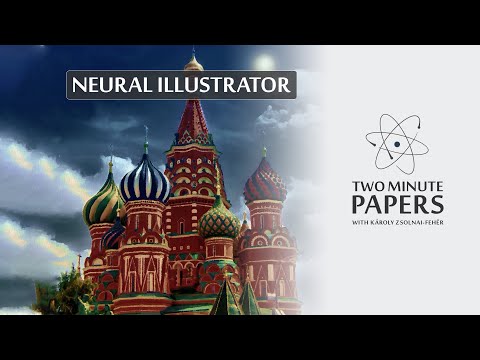
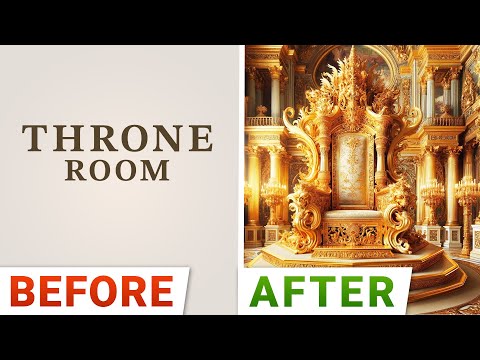
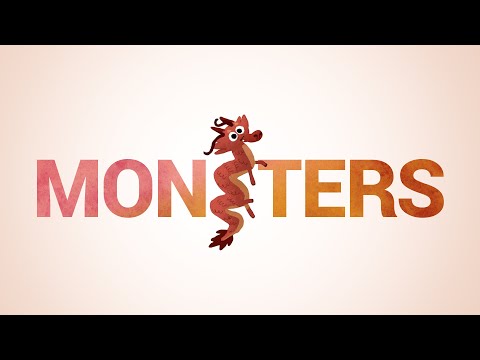
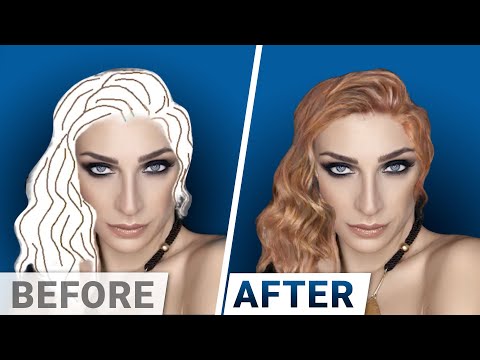
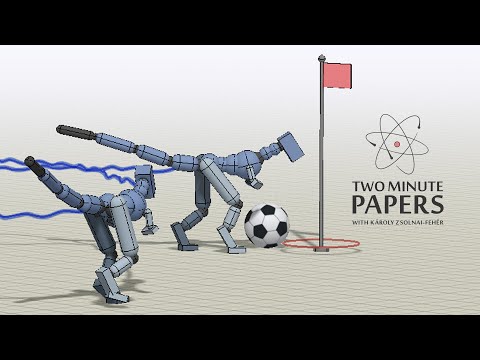