This Fools Your Vision | Two Minute Papers #241 | Summary and Q&A
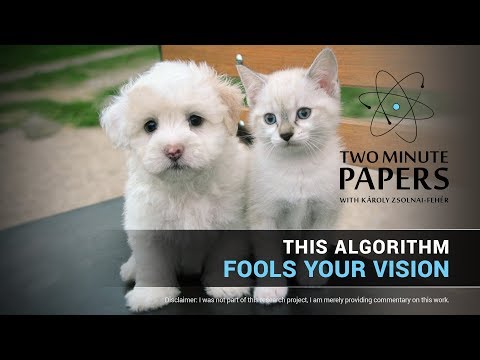
TL;DR
Neural networks can be manipulated by adding carefully crafted noise to an image, resulting in a misclassification. Surprisingly, humans can also be tricked into seeing an image differently due to shared features between architectures.
Key Insights
- 👊 Neural networks can be easily manipulated through adversarial attacks, reducing their accuracy significantly.
- 👊 Adversarial attacks on humans demonstrate shared features between machine and human vision systems.
- ❓ Modifying the noise generator model can better match the human visual system and deceive human perception.
- 👊 The architecture of a neural network influences the type of noise required for a successful adversarial attack.
- 🤨 Adversarial attacks highlight the need for robust AI systems and raise questions about the reliability of machine learning algorithms.
- 👊 The shared features between different neural network architectures make them susceptible to similar types of attacks.
- ❓ Some noise distributions that fool neural networks are also effective in manipulating human perception.
Transcript
Dear Fellow Scholars, this is Two Minute Papers with Károly Zsolnai-Fehér. Neural networks are amazing at recognizing objects when being shown an image, and in some cases, like traffic sign recognition, their performance can reach superhuman levels. But as we discussed in the previous episode, most of these networks have an interesting property whe... Read More
Questions & Answers
Q: How do adversarial attacks work on neural networks?
Adversarial attacks involve adding carefully crafted noise to an image, which causes misclassification by neural networks. The noise is generated based on the architecture of the network, targeting shared features.
Q: Can humans be fooled by adversarial attacks as well?
Yes, humans can also be tricked into perceiving an image differently due to shared features between neural network architectures. The modified image may retain some original features but can still be interpreted differently.
Q: What are the implications of adversarial attacks on both machines and humans?
Adversarial attacks highlight vulnerabilities in neural networks and raise concerns about the reliability of AI systems. Understanding how humans can also be manipulated by these attacks has implications for the study of human vision and perception.
Q: Are there universal adversarial attacks that can target any neural network?
Yes, the paper suggests that certain noise distributions can be effective across different neural network architectures. If an attack works on multiple networks, it is likely to work on other unseen networks as well.
Summary & Key Takeaways
-
Neural networks can be easily fooled by adding small changes to an image, causing them to misclassify it.
-
A recent algorithm performs an adversarial attack on humans, making them see a cat as a dog by adding noise.
-
Despite retaining cat-specific features, the modified image is perceived as a dog due to shared features between neural network architectures.
Share This Summary 📚
Explore More Summaries from Two Minute Papers 📚
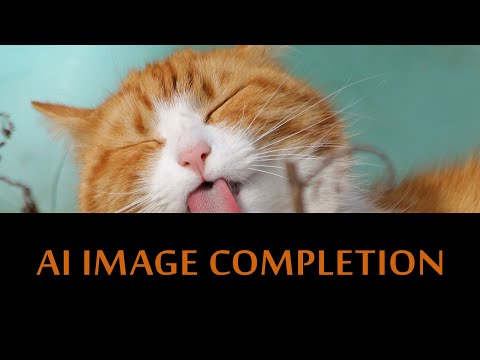
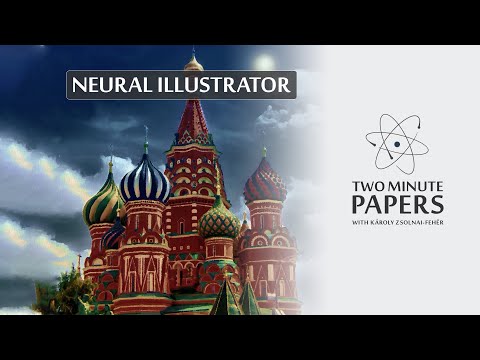
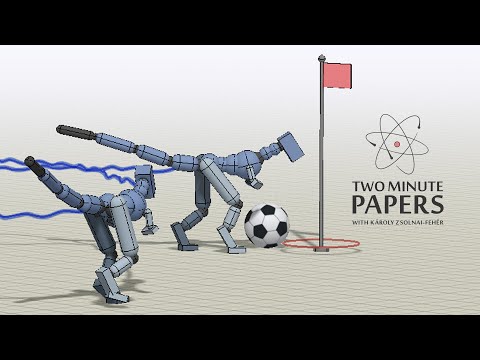
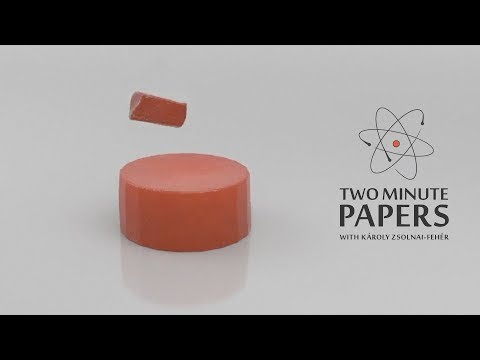
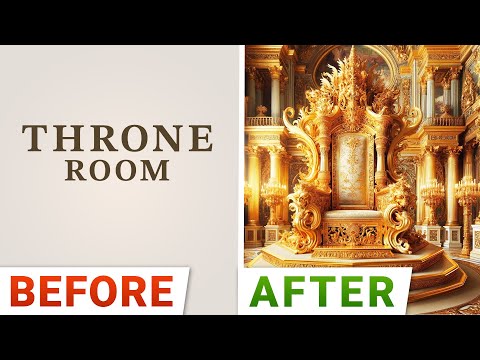
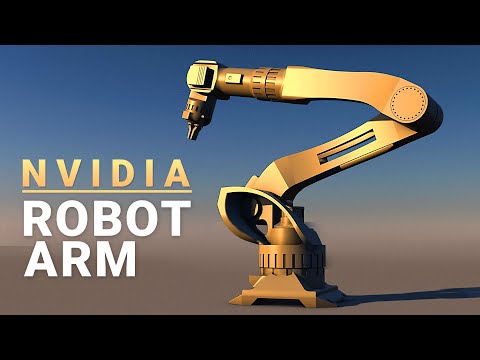