Terrain Generation With Deep Learning | Two Minute Papers #208 | Summary and Q&A
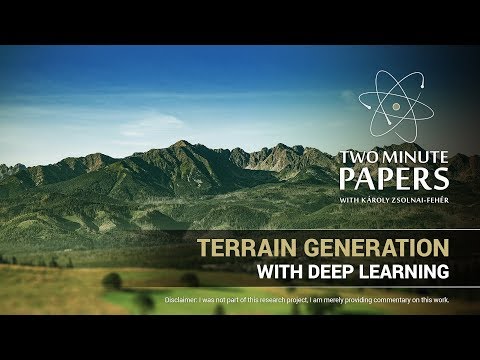
TL;DR
Neural networks can now generate high-resolution 3D models of terrains, benefiting computer games and the motion picture industry.
Key Insights
- ✋ Neural network-based techniques can generate high-resolution 3D models of terrains for virtual worlds.
- ❓ Procedural terrain generation can be improved and made more efficient using neural networks.
- 🚗 Auto-fill and physical manipulations like erosion are convenient features of the neural network-based terrain generation.
- 🔠 Sparse input may result in grid artifacts, but this limitation can be overcome through fine-tuning.
- 😘 The computational cost of the neural network for erosion is much lower compared to traditional simulation methods.
- 👾 This technique has the potential to greatly benefit industries like computer games and motion pictures.
- 👻 The neural network allows for real-time refinement of sketches and rapid generation of high-quality final results.
Transcript
Dear Fellow Scholars, this is Two Minute Papers with Károly Zsolnai-Fehér. We have recently witnessed the emergence of neural network-based techniques that are able to synthesize all sorts of images. Our previous episode was about NVIDIA's algorithm that created high resolution images of imaginary celebrities that was a really cool application of G... Read More
Questions & Answers
Q: How do neural networks generate high-resolution 3D models of terrains?
The neural network is trained to learn the correspondence between terrain images and input drawings. By sketching a draft of a landscape, the algorithm can generate a high-quality 3D model of the terrain.
Q: What are the benefits of using neural network-based terrain generation?
This technique allows for efficient and rapid terrain generation, eliminating the need for extensive manual work. It also offers conveniences like auto-fill for erased subregions and the ability to perform physical manipulations like erosion.
Q: What limitations does this technique have?
Sparse input may lead to unpleasant grid artifacts in the generated terrain. However, the researchers have addressed this issue by fine-tuning the neural network's training.
Q: How does the computational cost compare between the neural network and traditional simulation for erosion?
The neural network can perform erosion simulations almost instantaneously, while a traditional simulation may take up to a minute. The computational cost of the neural network for erosion is significantly lower.
Summary & Key Takeaways
-
Neural network-based techniques can generate all sorts of images, and now researchers aim to adapt these networks for terrain generation in virtual worlds.
-
By training the neural network to learn how an image of a terrain corresponds to input drawings, it can generate high-quality 3D models of terrains based on sketches.
-
The process is efficient and allows for refining drawings in real-time, automatically filling erased subregions, and performing physical manipulations like erosion.
Share This Summary 📚
Explore More Summaries from Two Minute Papers 📚
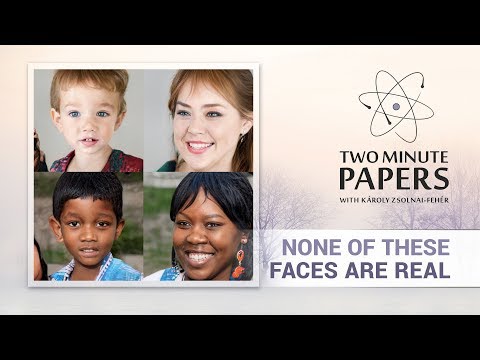
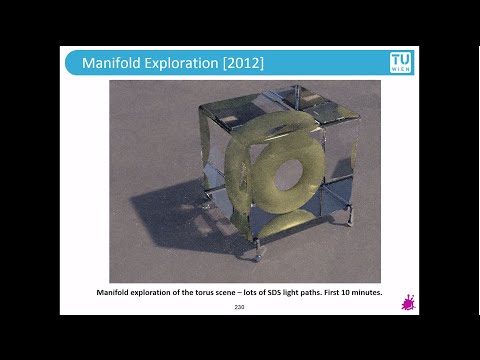
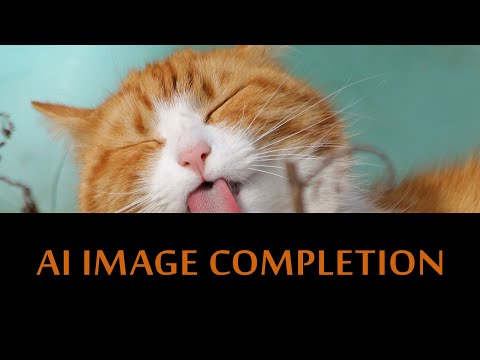
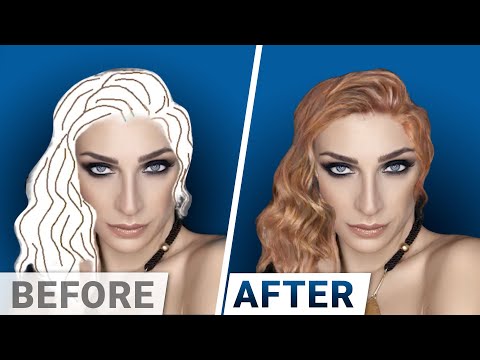
![Artificial Superintelligence [Audio only] | Two Minute Papers #29 thumbnail](https://i.ytimg.com/vi/08V_F19HUfI/hqdefault.jpg)
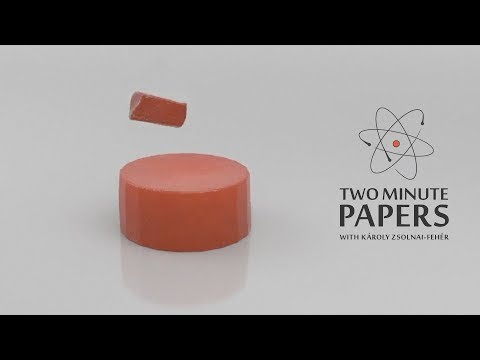