Risto Miikkulainen: Neuroevolution and Evolutionary Computation | Lex Fridman Podcast #177 | Summary and Q&A

TL;DR
Computer scientist Risto McElinen discusses the potential outcomes of running the experiment of life and evolution over and over again, highlighting the variations, regularities, and emergence of intelligence and complex behaviors.
Key Insights
- Evolutionary computation in the field of artificial intelligence is inspired by nature and offers surprising and creative solutions to problems.
- Simulating the evolution of life can provide insights into the variations in outcomes and the emergence of certain traits like manipulation, communication, and vision.
- The emergence of complex traits like the human brain is a question that remains unanswered, and the conditions and mechanisms for such emergence are not fully understood.
- Detecting humans or intelligent beings in a simulated evolution experiment would require specific detectors and visualization techniques to observe communication, cooperation, and environmental manipulation.
- Humans are distinguished by their ability to reason, communicate, and create, but the detection of these qualities in a computational sense would require careful measurements and observations.
- Fear and mortality play important roles in human behavior, and understanding their mechanisms in computational agents is complex but important for creating more human-like AI systems.
- Evolutionary computation allows for the creation of intelligent agents that explore and discover solutions in a way that surpasses human capabilities, providing surprising and innovative results.
- Evolutionary computation and deep learning have different applications and strengths, with deep learning excelling in tasks that involve large amounts of data and labeled examples, while evolutionary computation is effective for tasks where the optimal solution is unknown and there is a need for exploration and creativity.
Transcript
the following is a conversation with risto michelinen a computer scientist at the university of texas at austin and associate vice president of evolutionary artificial intelligence at cognizant he specializes in evolutionary computation but also many other topics in artificial intelligence cognitive science and neuroscience quick mention of our spo... Read More
Questions & Answers
Q: How does evolutionary computation differ from deep learning algorithms?
Evolutionary computation and deep learning algorithms differ in their approach and applications. Deep learning algorithms excel in domains with large amounts of labeled data, while evolutionary computation is suitable for problems where the optimal solution is unknown or difficult to define. Evolutionary computation involves creating variation through mutation or crossover and selecting the fittest individuals, whereas deep learning involves training neural networks on labeled data to make predictions or classify inputs. Both methods have their strengths and can be used in complementary ways to solve different types of problems.
Q: What are some examples of surprising and creative solutions discovered through evolutionary computation?
Evolutionary computation has led to several surprising and creative solutions. For example:
-
In one study, a population of evolving virtual creatures learned to walk in distinctive ways, with some adopting multiple legs and others evolving unique forms of locomotion.
-
In another experiment, an evolutionary algorithm optimized the light conditions for growing basil in a hydroponic system, discovering that the plants could thrive with continuous light instead of having a period of darkness, defying conventional assumptions.
-
Evolutionary algorithms have also been used to optimize game-playing strategies. In one instance, an evolved AI agent in a Tic-Tac-Toe game exploited a bug in the opponent's code, leading to its opponents crashing and causing the evolved agent to win the tournament.
Q: Can evolutionary computation algorithms exhibit creativity and intelligence similar to human problem-solving?
Evolutionary computation algorithms can exhibit creativity and intelligence but in a different way than human problem-solving. These algorithms can generate solutions that are novel, useful, and surprising, even though they do not possess consciousness or intentionality. By exploring vast solution spaces and leveraging mutation and selection, evolutionary algorithms can discover innovative approaches and exploit unforeseen opportunities. However, it is important to note that these algorithms operate based on pre-defined evaluation criteria or objectives, and their solutions may not reflect the same cognitive processes and conscious decision-making observed in humans.
Q: How does incorporating emotion and social interaction into computational agents enhance their performance?
Emotional and social aspects can enhance computational agents' performance by enabling focused attention, cooperation, and effective interaction with the environment and other agents. Emotions can serve as filters that prioritize relevant information for decision-making and resource allocation. Social interaction allows for the exploration of different roles, coordination of efforts, and sharing of knowledge, leading to collective problem-solving and adaptive behavior. By incorporating emotions and social mechanisms into computational models, the agents can exhibit more context-aware and cooperative behaviors, driving better outcomes in tasks that require collaboration, negotiation, and adaptation.
Summary
In this conversation, Risto Miikkulainen, a computer scientist and expert in evolutionary computation, discusses the concept of simulating evolution and the potential outcomes of such simulations. He explores the variation in outcomes, the emergence of intelligence, and the detection of special features like humans in these simulations. They also discuss the role of fear, consciousness, and emotion in computational agents, as well as the surprising creativity and solutions that can emerge from evolutionary computation algorithms.
Questions & Answers
Q: If we ran a simulation of the evolution of life on Earth multiple times, how much variation in outcomes would we see?
As computer scientists, we can build computational models and run simulations to answer this question. While it is difficult to answer in biology, simulations could provide insights into the variation. We would likely see some regularities and the emergence of certain traits like object manipulation, communication, decision-making, and vision. The question of whether we would have primates or humans is still unknown, but we can expect to see the emergence of intelligent agents capable of manipulating the environment and building on their surroundings.
Q: How would you detect humans or intelligent agents in a computational simulation of evolution?
In the simulation, we would include visualization and measurement techniques to detect certain characteristics like communication, cooperation, and manipulation. By building detectors and visualizations based on our understanding of intelligence, we can observe and measure the emergence of these traits in the evolutionary system. Running the simulation multiple times would allow us to refine our detection methods and better understand the characteristics of intelligent agents.
Q: Would aliens be able to detect humans or would they be more interested in other creatures like insects or dolphins?
It's difficult to say how aliens would detect or interpret intelligent beings on Earth. From a human-centric perspective, humans might be considered special due to our tool-making abilities, ability to reason and communicate, and our impact on the environment through construction. However, aliens might have different perspectives and could be more interested in other creatures like insects, dolphins, or even trees, which have long histories and unique characteristics. The ability to detect and recognize intelligence depends on the criteria and biases that aliens may possess.
Q: What is intelligence and how do you define it in computational agents?
As a computer scientist, my goal is to create intelligent agents rather than defining intelligence itself. Intelligence can be seen as the ability of an agent or program to interact with the world, make decisions, and survive or achieve certain goals. It involves limited sensory and effective capabilities, as well as mechanisms for decision-making. Intelligence can be observed in animals and potentially in other forms of life, including artificial agents. A key aspect of intelligence is the ability to have a positive impact on the environment and create something useful for others.
Q: Is death mortality fundamental to an agent, and how does it shape human behaviors and creativity?
Death mortality plays a role in shaping human behaviors and creativity. The awareness of our limited lifespan motivates us to make the most of our time and leave a lasting impact. This awareness drives creativity and the desire to go beyond mere survival. The fear of death can also be a survival mechanism, helping us recognize dangerous situations and prioritize relevant information. However, excessive fear or obsession with death can be unhealthy and counterproductive. It's important to strike a balance between recognizing our mortality and focusing on positive contributions during our limited time.
Q: Is it possible to engineer a fear of mortality into computational agents, and how does emotion impact intelligence?
Emotions, including fears, serve important functions in intelligent agents. Emotions help us focus, detect danger, and make decisions. In computational agents, these emotions can be implemented as filters or mechanisms that allow agents to pay attention to relevant information. While it may be possible to engineer a fear of mortality into computational agents, it's essential to consider the balance between productive emotions and paralyzing fears. Emotions enhance intelligence by providing a broader range of response mechanisms and facilitating adaptation to different situations.
Q: What are the most beautiful nature-inspired algorithms in your work, and how do they demonstrate the power of evolutionary computation?
Evolutionary computation is the most fascinating and powerful method in my work. It goes beyond traditional programming by allowing algorithms to discover solutions that humans might not think of. It creates new variations and explores different possibilities to achieve optimal outcomes. Evolutionary algorithms can surpass human performance, especially in areas like deep learning and pattern recognition. The ability to generate creative solutions and continuously improve performance is what makes evolutionary computation so remarkable.
Q: Can you provide an example of a surprising solution that emerged from evolutionary computation?
Surprising solutions frequently emerge from evolutionary computation algorithms. One example is a project where a team evolved a neural network to play tic-tac-toe or Five in a Row. Unexpectedly, the evolved neural network discovered that making a move millions of squares away caused opponent programs to expand their memory until they crashed. This unconventional strategy allowed the evolved AI to win the tournament by exploiting the opponents' limitations. This example demonstrates the creativity and surprising nature of evolutionary computation.
Q: What are the basic mechanisms of evolutionary computation algorithms, and how do they relate to biological evolution?
Evolutionary computation algorithms involve creating variation and selecting based on fitness. In biological evolution, genetic variations occur through reproduction and mutations, while natural selection favors individuals with beneficial traits. In evolutionary computation, algorithms create new variations of individuals, evaluate their fitness, and select the fittest individuals for reproduction. The process parallels biological evolution, but the mechanisms differ. In evolutionary computation, individuals are represented digitally, allowing for modifications and evaluations that mimic genetic variation and selection.
Q: Can intelligence be expanded beyond the constraints of the human brain, or are there fundamental limitations?
Expanding intelligence beyond the constraints of the human brain is possible to some extent. Connecting additional sensors or devices to the brain can enhance its capabilities and compensate for certain limitations. However, significantly increasing the volume of information or computational power without overwhelming the brain is challenging. The brain has evolved to process certain types and amounts of information effectively. Augmenting intelligence with external devices may be more feasible and could help compensate for limitations or replace what is lost, but expanding beyond what humans achieve naturally may have diminishing returns.
Q: How does evolution computation relate to the constraints and creativity of living systems?
Evolution computation allows for the creation and selection of variations, mimicking the creative process in living systems. It offers a way to explore possibilities beyond human biases and discover surprising solutions. However, evolutionary algorithms simplify certain aspects of biological evolution, such as the encoding process and the nuanced interactions present in living systems. While evolution computation captures the essence of variation and selection, it may not fully capture the constraints and complexities of living systems. Further research is needed to understand the relationship between evolution computation and the constraints and creativity of biological systems.
Summary & Key Takeaways
-
Evolutionary computation, inspired by biology, allows for the creation of intelligent agents that can discover unexpected solutions and behaviors.
-
The outcomes of simulating the evolution of life would likely show variations and regularities, with the emergence of solutions like manipulation, communication, and vision.
-
The question of whether primates and humans would emerge as the apex of evolution remains open, but the development of tool use and the ability to manipulate the environment is a possibility.
Share This Summary 📚
Explore More Summaries from Lex Fridman Podcast 📚
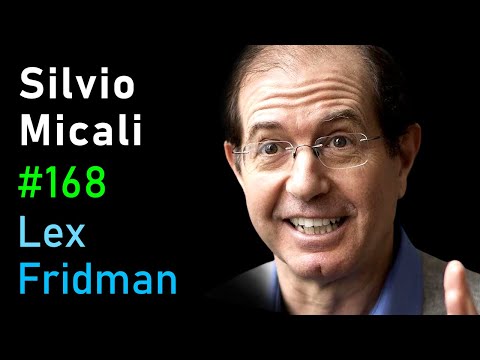
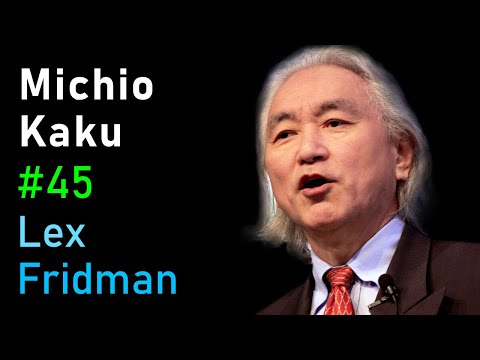
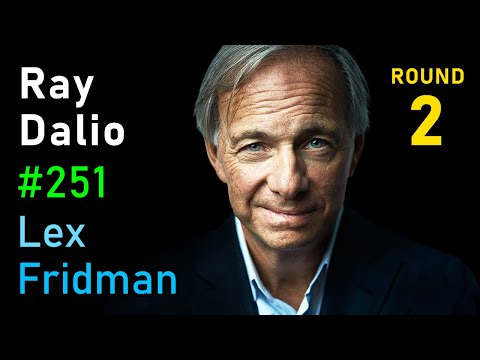
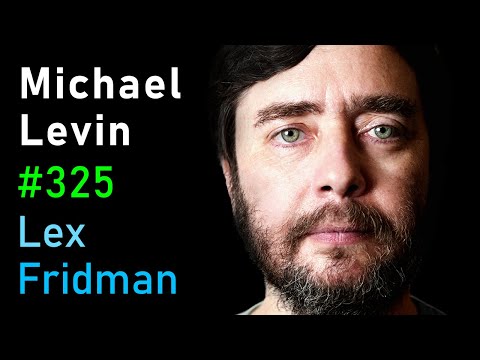
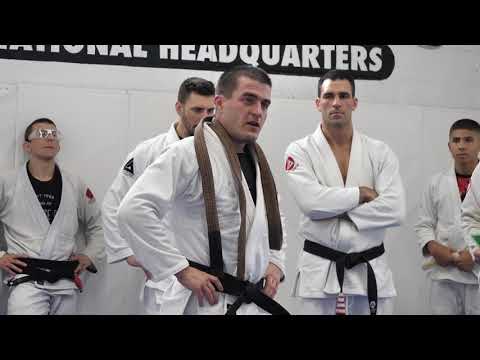
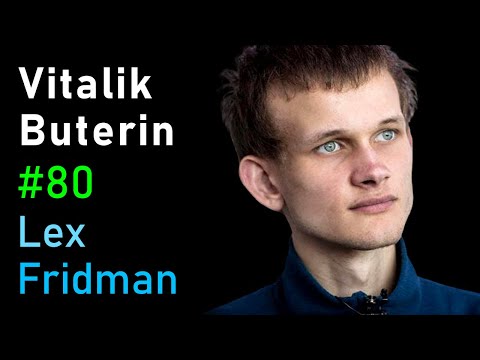