Google DeepMind's Deep Q-Learning & Superhuman Atari Gameplays | Two Minute Papers #27 | Summary and Q&A
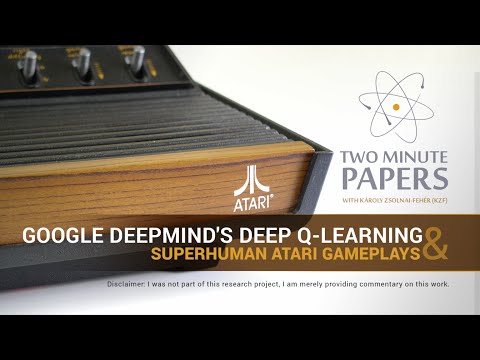
TL;DR
Deep Q-Learning is a combination of convolutional neural networks and reinforcement learning algorithms that enables machines to learn how to play games like humans, achieving superhuman performance.
Key Insights
- 🎮 Deep Q-Learning combines convolutional neural networks and reinforcement learning, allowing machines to learn how to play games like humans.
- ⌛ The algorithm initially performs poorly but improves over time, matching the skill level of adept players.
- 👾 Deep Q-Learning has been successfully applied to various games, achieving superhuman performance.
Transcript
Dear Fellow Scholars, this is Two Minute Papers with Károly Zsolnai-Fehér. This one is going to be huge, certainly one of my favorites. This work is a combination of several techniques that we have talked about earlier. If you don't know some of these terms, it's perfectly okay, you can remedy this by clicking on the popups or checking the descript... Read More
Questions & Answers
Q: What is Deep Q-Learning?
Deep Q-Learning is an approach that combines convolutional neural networks and reinforcement learning algorithms, allowing machines to learn how to play games by processing images and making decisions on actions.
Q: How does Deep Q-Learning improve its gameplay?
Initially, the algorithm performs poorly, but over time, it learns to play the game and matches the skill level of an adept player. It can even discover unique strategies such as digging tunnels to win the game.
Q: Can Deep Q-Learning be applied to other games?
Yes, Deep Q-Learning has been applied to various games, and the algorithm's performance is measured in relation to human players. A score above 100% indicates superhuman performance.
Q: What are the potential future directions for Deep Q-Learning?
Two unexplored directions are the incorporation of long-term memory into the algorithm and its ability to generalize knowledge to other similar tasks.
Summary & Key Takeaways
-
Deep Q-Learning is a technique that combines convolutional neural networks and reinforcement learning algorithms to enable machines to learn how to play games.
-
Initially, the algorithm performs poorly, but with time, it becomes adept at playing the game, even discovering innovative strategies not known to humans.
-
Scientists have also applied Deep Q-Learning to 3D games, where machines can learn to drive on ideal racing lines and surpass human performance.
Share This Summary 📚
Explore More Summaries from Two Minute Papers 📚
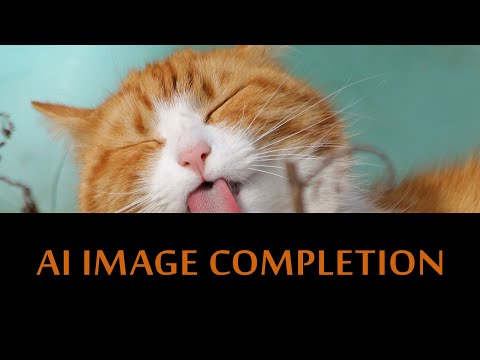
![Artificial Superintelligence [Audio only] | Two Minute Papers #29 thumbnail](https://i.ytimg.com/vi/08V_F19HUfI/hqdefault.jpg)
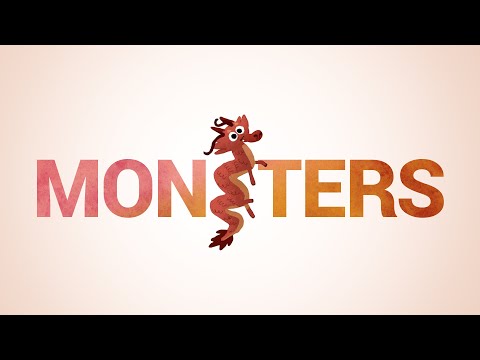
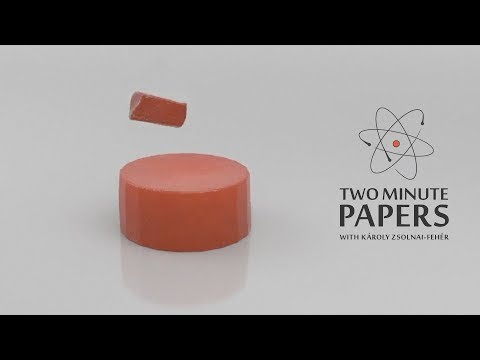

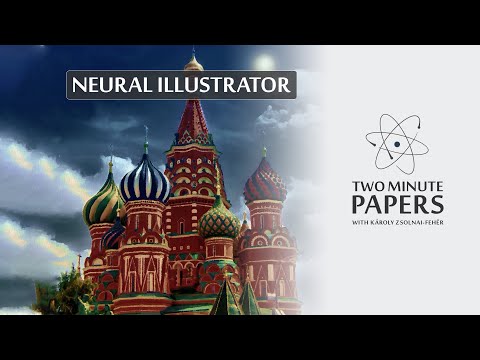