Finally, Differentiable Physics is Here! | Summary and Q&A

TL;DR
This paper introduces differentiable programming for physical simulations, allowing systems to compute required forces and angles to achieve desired outcomes.
Key Insights
- 👻 Differentiable programming allows for the computation of inputs required to achieve desired outcomes in physical simulations.
- 🚶 The system can be applied to various problems, such as teaching objects to walk and manipulating fluid simulations.
- 😉 The ability to manipulate ink simulations to form complex patterns showcases the power and creativity of differentiable programming.
- 💨 The system's capacity to distort images in a way that neural networks perceive differently demonstrates its versatility.
- 👻 The availability of the source code allows for further exploration and experimentation with differentiable programming.
- 🎮 The system's potential applications include faster problem-solving in video games and novel problem definitions.
- 📽️ Weights & Biases, a project tracking tool, supports the development of deep learning projects and was essential in the implementation of the discussed paper.
Transcript
Dear Fellow Scholars, this is Two Minute Papers with Károly Zsolnai-Fehér. A few episodes ago, we discussed a new research work that performs something that they call differentiable rendering. The problem formulation is the following: we specify a target image that is either rendered by a computer program, or even better, a photo. The input is a pi... Read More
Questions & Answers
Q: What is differentiable programming for physical simulations?
Differentiable programming for physical simulations refers to a system that can compute the forces and angles required to achieve desired outcomes in simulations. It allows for specifying an end state and automatically calculates the necessary inputs to reach that state.
Q: What are some examples of applications for differentiable programming in simulations?
Some examples include teaching objects to navigate obstacles, manipulating fluid simulations to take specific shapes, and distorting images in ways that neural networks perceive differently.
Q: How robust is the 3D simulation taught to walk?
The 3D simulation is taught to walk so robustly that it can even withstand a few extra particles in its path. This demonstrates the system's ability to handle complex and challenging scenarios.
Q: Where can one find the full source code for this work?
The full source code for this work is available, and interested individuals can find it in the video description.
Summary & Key Takeaways
-
The paper presents a differentiable programming system that can compute the forces and angles required to achieve a desired outcome in physical simulations.
-
The system is able to teach a gooey object to walk and withstand obstacles, as well as manipulate fluid simulations to take specific shapes.
-
It demonstrates the ability to manipulate ink simulations to form complex patterns and distort images in a way that neural networks perceive differently.
Share This Summary 📚
Explore More Summaries from Two Minute Papers 📚
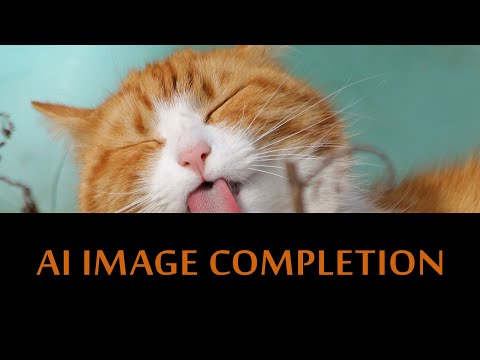
![Artificial Superintelligence [Audio only] | Two Minute Papers #29 thumbnail](https://i.ytimg.com/vi/08V_F19HUfI/hqdefault.jpg)
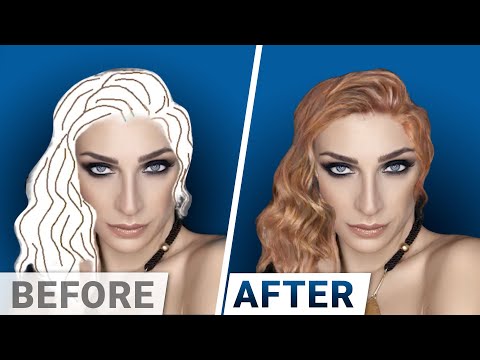
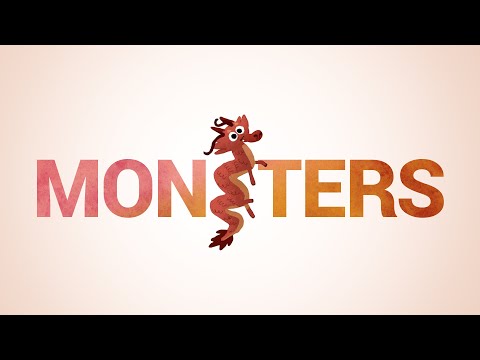
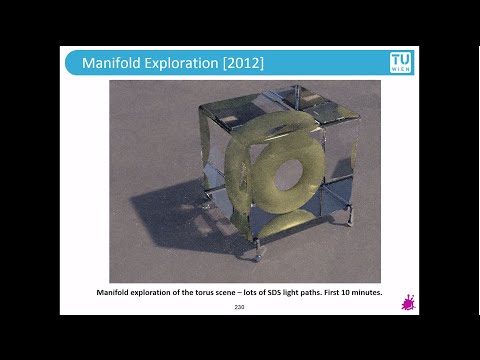
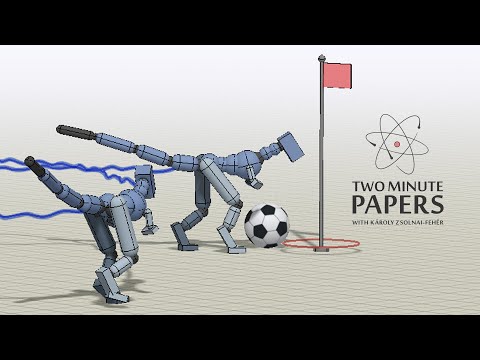