Deep Learning and Cancer Research | Two Minute Papers #64 | Summary and Q&A
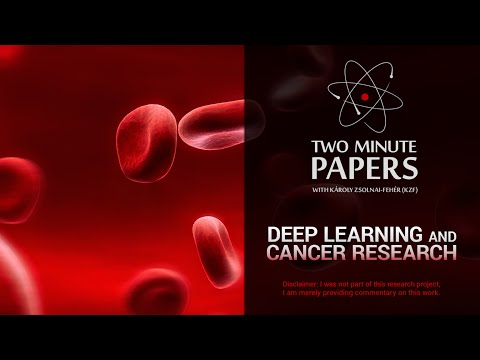
TL;DR
A new label-free technique that uses deep learning to detect cancer cells without damaging the samples shows high accuracy and potential for early detection.
Key Insights
- 😒 The label-free technique uses images of cells to detect cancer cells accurately without damaging the samples.
- ⏮️ Previous techniques involving chemicals were unreliable and destroyed cells, hindering later analysis.
- 🙂 Morphological features, refractive indices, light absorption, and scattering properties are important features for cancer detection.
- ♋ Deep learning and neural networks help identify the relationship between these features and cancer cells.
- ☠️ Early detection of cancer is crucial for higher survival rates.
- 🥶 Non-intrusive label-free techniques, like the one proposed, have the potential to revolutionize cancer detection.
- ✋ The proposed technique shows significantly higher accuracy and consistency than previous methods.
Transcript
Dear Fellow Scholars, this is Two Minute Papers with Károly Zsolnai-Fehér. Let's try to assess the workflow of this piece of work in the shortest possible form. The input is images of cells, and the output of the algorithm is a decision that tells us which one of these are cancer cells. As the pipeline of the entire experiment is quite elaborate, w... Read More
Questions & Answers
Q: How does the label-free technique for cancer cell detection work?
The technique uses images of cells to analyze refractive indices, light absorption, scattering properties, and cell morphology. Neural networks are trained to learn the relation between these features and cancer cells, enabling accurate detection without damaging the samples.
Q: What are the advantages of this label-free technique over previous techniques?
Unlike previous techniques that involved adding chemicals to blood samples, this technique is non-intrusive and does not destroy cells. It also shows significantly improved accuracy and consistency in cancer cell detection.
Q: How can deep learning contribute to early detection of cancer?
Early detection of cancer is crucial for higher survival rates. Deep learning, combined with image analysis techniques, can aid in the early identification of cancer cells, as shown by the chart displaying ovarian cancer survival rates based on detection timing.
Q: What role do different professionals play in the fight against cancer?
Engineers create instruments to analyze blood samples, doctors identify important features for cancer detection, and computer scientists work on finding relationships between these features and illnesses. Collaboration among these fields has contributed to significant progress in cancer detection.
Summary & Key Takeaways
-
The input for this technique is images of cells, and the output is a decision on which cells are cancerous.
-
Previous techniques involved adding chemicals to blood samples, which were not reliable and destroyed cells, making it impossible to check samples later.
-
The proposed label-free technique uses features like refractive indices, light absorption and scattering properties, and cell morphology, combined with neural networks, to locate cancer cells accurately and consistently.
Share This Summary 📚
Explore More Summaries from Two Minute Papers 📚
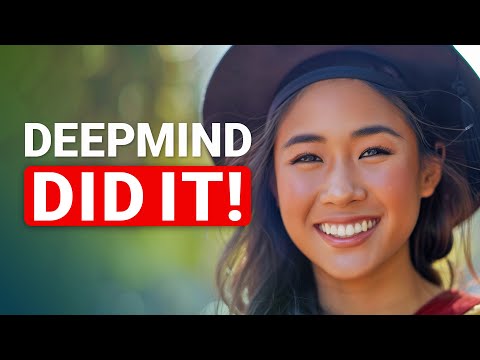
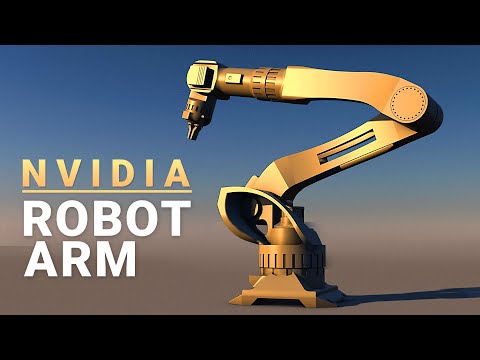
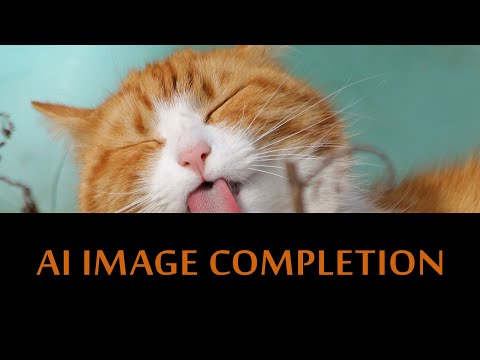
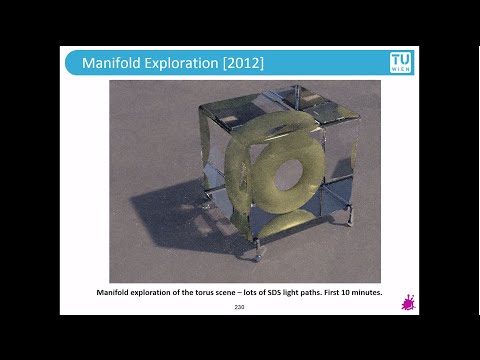
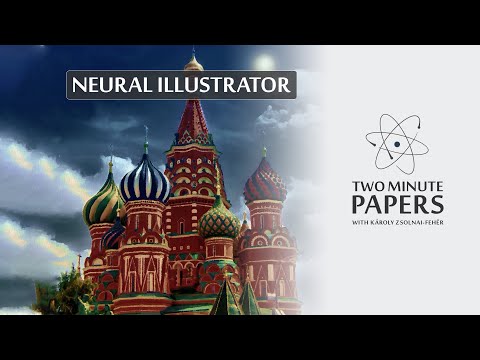
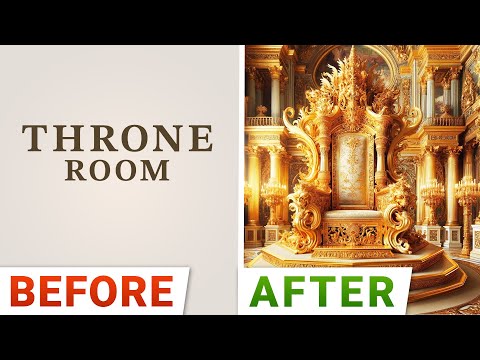