David Ferrucci: IBM Watson, Jeopardy & Deep Conversations with AI | Lex Fridman Podcast #44 | Summary and Q&A
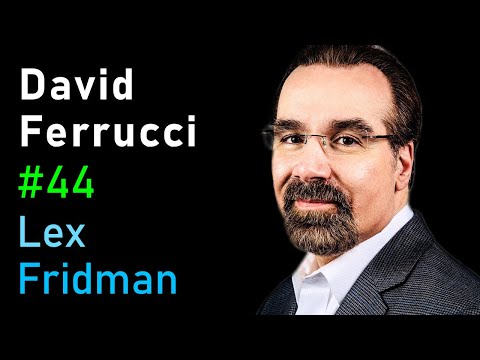
TL;DR
David Ferrucci discusses the differences between biological systems and computer systems, the challenges of creating AI that can understand and communicate like humans, and the potential of AI in democratizing information access.
Key Insights
- ⚾ AI systems can learn patterns and make predictions based on data, but understanding and explaining the reasoning process is crucial for effective communication with humans.
- 💁 Human intelligence relies on frameworks and shared knowledge, while AI intelligence focuses on pattern recognition and prediction. Bridging these two forms of intelligence is important for collaboration and shared understanding.
- 🤔 AI systems can help overcome human biases by providing alternative perspectives and encouraging critical thinking.
- ❓ Acquiring and communicating frameworks is a challenge for AI systems, but it is essential for human-AI collaboration and understanding.
Transcript
following is a conversation with David Ferrucci he led the team that built Watson the IBM question-answering system that beat the top humans in the world at the game of Jeopardy for spending a couple hours of David I saw a genuine passion not only for abstract understanding of intelligence but for engineering it to solve real-world problems under r... Read More
Questions & Answers
Q: How does the process of learning and understanding work in AI systems like Watson?
AI systems like Watson use machine learning techniques to learn patterns in data and make predictions based on that learning. They can acquire knowledge and connect it to frameworks to interpret and reason about the data. However, the challenge lies in explaining and communicating the reasoning process, which requires the integration of both specific knowledge and broader frameworks.
Q: Why is it important to bridge the gap between human and AI understanding?
Bridging the gap between human and AI understanding is crucial for effective communication and collaboration. Humans rely on intuition, emotion, and shared frameworks to interpret information, while AI systems focus on pattern recognition and prediction. By enabling AI systems to acquire and communicate frameworks, we can enhance human-AI collaboration and overcome biases and limitations in human reasoning.
Q: How can AI systems help humans overcome biases and make more objective decisions?
AI systems can assist humans in overcoming biases by providing alternative perspectives and reasoning processes. By analyzing large amounts of data and detecting hidden patterns, AI can challenge our assumptions and encourage critical thinking. Through collaboration, humans can learn from AI systems and improve their decision-making by considering multiple viewpoints and assessing the logic behind them.
Q: What are the challenges in creating AI systems that can understand and communicate like humans?
The challenges include acquiring frameworks for interpreting information, linking specific knowledge to those frameworks, and explaining the reasoning process to humans. AI systems need to learn not just specific facts and patterns but also the underlying principles and values that shape human understanding. The goal is to create AI systems that can effectively communicate and reason with humans in a way that aligns with human intelligence.
More Insights
-
AI systems can learn patterns and make predictions based on data, but understanding and explaining the reasoning process is crucial for effective communication with humans.
-
Human intelligence relies on frameworks and shared knowledge, while AI intelligence focuses on pattern recognition and prediction. Bridging these two forms of intelligence is important for collaboration and shared understanding.
-
AI systems can help overcome human biases by providing alternative perspectives and encouraging critical thinking.
-
Acquiring and communicating frameworks is a challenge for AI systems, but it is essential for human-AI collaboration and understanding.
-
AI systems should be designed to understand the context, values, and reasoning processes that shape human intelligence in order to enhance collaboration and decision-making.
Summary
In this conversation with David Ferrucci, the creator of Watson, the IBM question-answering system that won Jeopardy, they discuss the difference between biological and computer systems, the nature of intelligence, the challenge of explaining reasoning, the role of emotions in decision-making, encoding knowledge, and the potential for machines to learn and communicate with humans.
Questions & Answers
Q: What is the difference between biological and computer systems?
David Ferrucci suggests that while there may be differences in implementation, there may not be a substantial difference in their capabilities. He believes that the ability to describe and understand a process, even if it is an intelligent process, could be the defining factor.
Q: Is the biological implementation of intelligence fundamentally more capable than what we can build with computers?
Ferrucci argues that the capabilities of biological and computer systems may not be so different. He suggests that the goal of recreating biological intelligence should not be the primary focus, but rather understanding it to diagnose and treat issues, and to determine what we want out of intelligence.
Q: How can we understand intelligence beyond the ability to predict accurately and consistently?
Ferrucci explains that beyond prediction, the ability to articulate and explain the reasoning process is a crucial aspect of intelligence. He believes that the ability to communicate and relate to each other is essential.
Q: Are the flaws in human intelligence, such as prejudice, forgetfulness, and fear, hindrances to intelligence or essential components?
Ferrucci argues that these flaws can be seen as both hindrances and essential components of human intelligence. While they may affect objectivity, they also serve a purpose in survival and quick decision-making. However, he notes that humans are capable of both quick and deep thinking.
Q: How does reasoning through logic play a role in human intelligence?
Ferrucci suggests that reasoning through logic is harder for individuals and requires training, but it is a skill that can be developed. Humans are capable of both quick and deep thinking, with the ability to reason and make more objective decisions.
Q: How do you define intelligence and its relationship to reasoning?
Ferrucci defines intelligence as the ability to accurately, precisely, rigorously reason; develop answers; and justify those answers in an objective way. The ability to predict accurately and consistently is a form of intelligence.
Q: What is needed to predict accurately?
Ferrucci explains that in order to predict accurately, an understanding of the world is necessary. Machines need to find functions and learn patterns using prior data, and the ability to find what's worth predicting is also important.
Q: Can computers learn to predict accurately with less data and training time?
Ferrucci suggests that machines can become smarter if they can predict accurately with less data and training time. Figuring out what's worth predicting is a sign of intelligence.
Q: What role do frameworks play in understanding and communicating intelligence?
Ferrucci emphasizes that frameworks are essential for interpreting and reasoning about the world. Humans share frameworks based on their shared experiences, and machines need to acquire and connect those frameworks to the data in order to communicate their understanding.
Q: How hard is it to encode and teach computers the frameworks that underlie human understanding?
Ferrucci admits that teaching computers the frameworks is challenging. While machines can learn patterns easily, acquiring and connecting the frameworks to the data requires more effort. The challenge lies in understanding and interpreting the meaning behind the data.
Takeaways
The conversation with David Ferrucci explores the differences between biological and computer systems, the nature of intelligence, and the challenges of explaining reasoning. It highlights the importance of understanding and communicating frameworks, as well as the difficulties in encoding and teaching computers to acquire these frameworks. Ultimately, the goal is to build machines that can predict accurately, reason, and communicate understanding with humans in terms of shared frameworks.
Summary & Key Takeaways
-
David Ferrucci, creator of IBM's Watson, discusses the challenge of creating AI systems that can solve real-world problems under constraints like humans.
-
He highlights the difference between biological and computer systems and the philosophical question of what sets humans apart from machines.
-
Ferrucci emphasizes the importance of understanding frameworks in intelligence, the need to communicate and explain reasoning, and the impact of biases and limitations on human and AI intelligence.
Share This Summary 📚
Explore More Summaries from Lex Fridman Podcast 📚
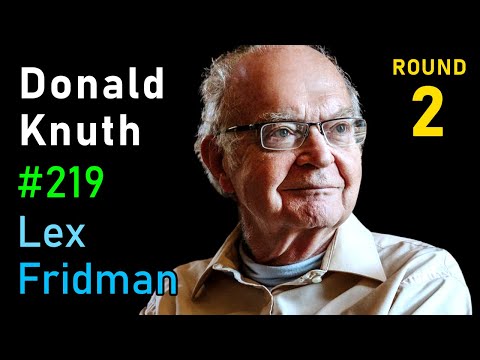


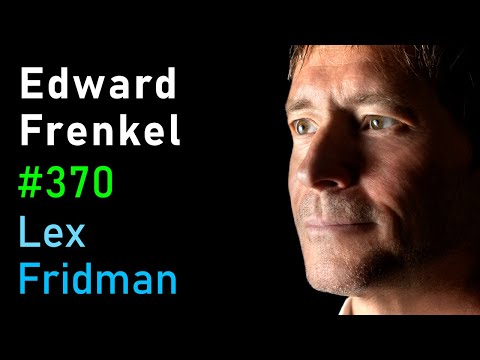

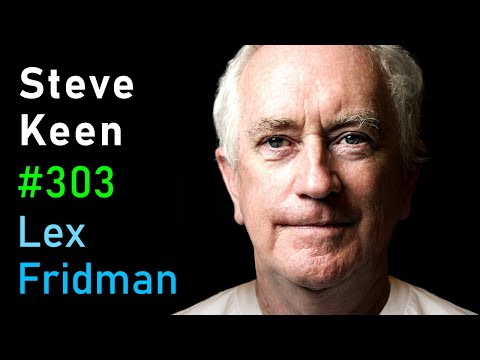