What Can We Learn From Deep Learning Programs? | Two Minute Papers #75 | Summary and Q&A
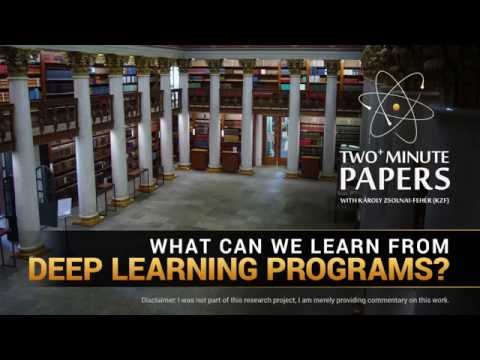
TL;DR
Deep learning papers are being rejected from computer vision conferences for not adding significantly to the existing knowledge, raising questions about the value of neural networks and scientific progress.
Key Insights
- 🏑 Neural networks can achieve impressive results but may not contribute significantly to knowledge in a field.
- 💁 Neural networks are not intuitively understandable due to their complexity and storage format.
- ❓ Attempts to insert human knowledge into neural networks can hinder their performance.
- 💨 Model compression offers a way to extract knowledge from neural networks by compressing their output into concise rules.
- 🤨 Efficient algorithms that lack interpretability raise questions about what defines scientific progress.
- 🎰 To achieve scientific progress, it is important to balance knowledge extraction and efficiency in machine learning algorithms.
- 🙈 Neural networks can be seen as a form of automated research, randomly experimenting and eventually producing concise rules to explain observations.
Transcript
Dear Fellow Scholars, this is Two Minute Papers with Károly Zsolnai-Fehér. I have recently been witnessing a few heated conversations regarding the submission of deep learning papers to computer vision conferences. The forums are up in arms about the fact that despite some of these papers showcased remarkably good results, they were rejected on the... Read More
Questions & Answers
Q: Why are deep learning papers being rejected from computer vision conferences?
Deep learning papers are rejected because although they achieve good results, they do not provide substantial advancements to the existing knowledge in the field.
Q: Why are neural networks not intuitively understandable?
Neural networks are complex and store information in a way that can take up several gigabytes. The best solutions often involve patterns and connections that are not easily interpretable by humans.
Q: Can we insert our knowledge into neural networks to improve their performance?
Attempts to forcefully insert human knowledge into neural networks often lead to worse results. Neural networks excel at learning patterns and making decisions based on observed data rather than explicit rule learning.
Q: How can model compression contribute to knowledge extraction from neural networks?
Model compression is a technique that aims to compress the information stored in neural networks into smaller, more understandable representations. By compressing the network's output into concise rules, it becomes possible to extract insights and knowledge from the model.
Summary & Key Takeaways
-
Some deep learning papers with impressive results are being rejected from computer vision conferences for not contributing enough to the existing knowledge.
-
Neural networks generate models that resemble the brain but are often not intuitively understandable to humans.
-
Neural networks are trained similarly to how language is learned, through exposure to correct examples rather than explicit rule learning.
Share This Summary 📚
Explore More Summaries from Two Minute Papers 📚
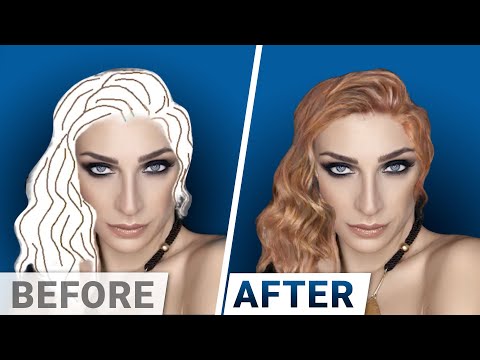
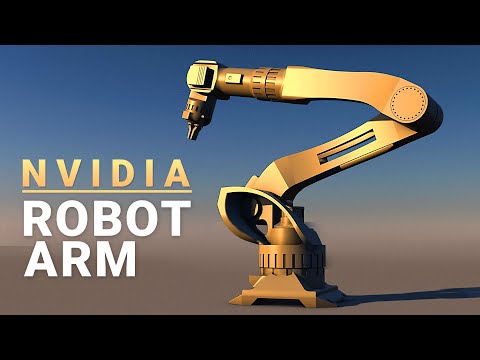
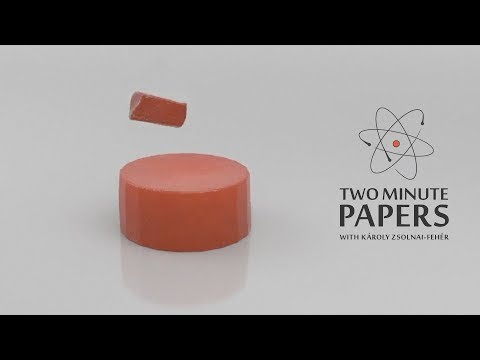
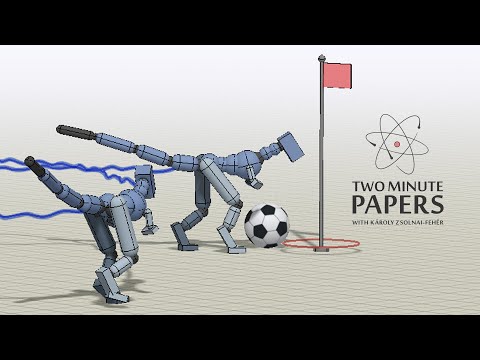
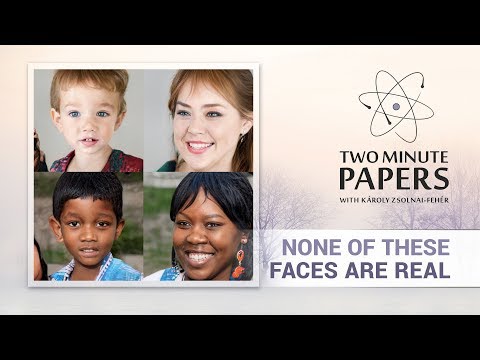
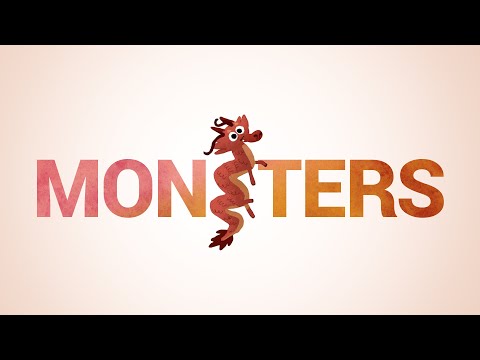