DeepMind solves protein folding | AlphaFold 2 | Summary and Q&A
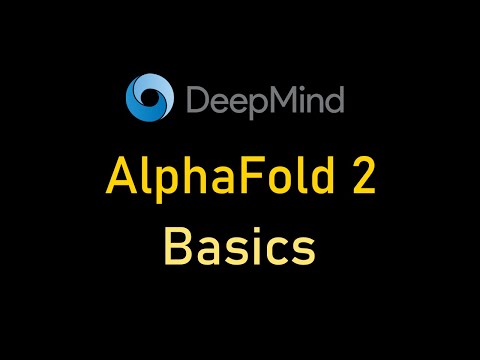
TL;DR
DeepMind's AlphaFold 2 has solved the 50-year-old grand challenge problem of protein folding, with the potential to revolutionize structural biology and drive advancements in medicine and other fields.
Key Insights
- 🙏 DeepMind's AlphaFold 2 has solved the 50-year-old grand challenge problem of protein folding, achieving impressive prediction performance comparable to experimental methods like x-ray crystallography.
- 👶 The breakthrough has significant implications for understanding protein function, identifying the causes of diseases, and designing new treatments.
- 🏑 AlphaFold 2's abilities can revolutionize structural biology, enabling researchers to rapidly determine protein structures and make discoveries in various scientific fields.
- 🔬 The potential impact of AlphaFold 2 extends beyond biology and medicine, with applications in materials science, bioengineering, bioinformatics, and biophysics.
- ✊ AlphaFold 2's success highlights the power of machine learning and computational methods in accelerating scientific advancements and addressing complex problems in various domains.
- ❤️🩹 The future implications of AlphaFold 2 include the advancement of end-to-end learning systems, the simulation of biological systems at a cellular or organ level, and even the exploration of artificial life.
Transcript
i think it's fair to say that this year 2020 has thrown quite a few challenges at human civilization so it's really nice to get some positive news about the truly marvelous accomplishments of engineering and science one was spacex i would argue launching a new era of space exploration and now a couple of days ago deepmind has announced that its sec... Read More
Questions & Answers
Q: What is the significance of DeepMind's breakthrough in protein folding?
DeepMind's AlphaFold 2 has achieved a solution to the long-standing problem of protein folding, offering great potential for understanding protein function, discovering new gene functions, and finding cures for diseases caused by misfolded proteins.
Q: How does AlphaFold 2 compare to the previous iteration, AlphaFold 1?
AlphaFold 2 has significantly improved upon AlphaFold 1's performance, achieving a score of 87, compared to the previous score of 58. This represents a substantial leap in computational prediction capabilities for protein structure.
Q: What is the potential impact of AlphaFold 2 in the field of medicine?
AlphaFold 2's ability to accurately predict protein structures could lead to the development of new treatments for diseases caused by misfolded proteins. It may also enable the design of proteins that fix misfolded proteins, opening up possibilities for drug development and tissue regeneration.
Q: How might AlphaFold 2 advance the field of structural biology?
AlphaFold 2's computational methods can dramatically increase the number of protein structures available for researchers to study and model. This can drive advancements in various areas, including materials science, bioengineering, and biophysics.
Q: What is the significance of DeepMind's breakthrough in protein folding?
DeepMind's AlphaFold 2 has achieved a solution to the long-standing problem of protein folding, offering great potential for understanding protein function, discovering new gene functions, and finding cures for diseases caused by misfolded proteins.
More Insights
-
DeepMind's AlphaFold 2 has solved the 50-year-old grand challenge problem of protein folding, achieving impressive prediction performance comparable to experimental methods like x-ray crystallography.
-
The breakthrough has significant implications for understanding protein function, identifying the causes of diseases, and designing new treatments.
-
AlphaFold 2's abilities can revolutionize structural biology, enabling researchers to rapidly determine protein structures and make discoveries in various scientific fields.
-
The potential impact of AlphaFold 2 extends beyond biology and medicine, with applications in materials science, bioengineering, bioinformatics, and biophysics.
-
AlphaFold 2's success highlights the power of machine learning and computational methods in accelerating scientific advancements and addressing complex problems in various domains.
-
The future implications of AlphaFold 2 include the advancement of end-to-end learning systems, the simulation of biological systems at a cellular or organ level, and even the exploration of artificial life.
-
This breakthrough may pave the way for machine learning systems to play a significant role in Nobel Prize-level discoveries, potentially transforming the traditional boundaries of scientific attribution.
Summary
This video discusses the recent breakthrough in protein folding achieved by DeepMind's AlphaFold system. It explains the significance of protein folding and its connection to diseases, and it explores the potential applications and future impact of this breakthrough in various fields including medicine, biology, agriculture, and materials science. The video also provides some insights into how AlphaFold works and highlights the important role of machine learning and attention mechanisms in its approach.
Questions & Answers
Q: What are the major accomplishments of the engineering and science field in 2020?
In 2020, SpaceX made significant strides in space exploration, while DeepMind announced the second iteration of the AlphaFold system, which has successfully tackled the grand challenge of protein folding prediction.
Q: What does it mean for AlphaFold to "solve" the protein folding challenge?
When we say AlphaFold has "solved" protein folding, it means that it has achieved prediction performance comparable to experimental methods like x-ray crystallography but at a faster and more affordable rate.
Q: How does AlphaFold's performance compare to its previous version and other competitors?
AlphaFold's second iteration achieved a score of 87 on the hardest class of proteins, which is a significant improvement from its score of 58 in the previous iteration. It also outperformed the closest competition by 26 points.
Q: How does this breakthrough in protein folding compare to other major achievements in the fields of structural biology and artificial intelligence?
Many people are comparing this breakthrough to the "ImageNet moment" in computer vision, as it is seen as a significant advancement in the field of artificial intelligence. In the life sciences, it is considered one of the most significant advancements in structural biology in the past two decades.
Q: What are some other major breakthroughs in the field of artificial intelligence?
Some other major breakthroughs in artificial intelligence include AlphaZero with reinforcement learning self-play, the application of transformers in natural language processing (GPT-3), the deployment of robots in various industries, and advancements in hardware engineering, such as Boston Dynamics' robot dog, Spot.
Q: What is the potential impact of AlphaFold's breakthrough in protein folding?
The ability to accurately predict protein structures could lead to a better understanding of gene functions and disease causes, potentially resulting in improved treatments and drugs. It could also have applications in agriculture, tissue regeneration, bio-materials, and advanced physics-based simulations of biological systems.
Q: How does AlphaFold's algorithm work?
While the details of AlphaFold 2 are not yet publicly available, it is clear that it utilizes attention mechanisms and transformers, following the trend in the deep learning field. It likely incorporates the multiple sequence alignment of evolutionary related sequences as part of the learning process and involves an iterative passing of learned information between different representations of the input data.
Q: Why is the protein folding problem considered challenging?
Protein folding is the process of going from the amino acid sequence to the 3D structure, and it is fascinating because a specific sequence typically corresponds to a unique structure. However, the number of possible combinations is astronomically large, making it a highly complex problem. Additionally, misfolding proteins are often the underlying cause of many diseases.
Q: How has experimental determination of protein structures been achieved in the past?
Experimental methods, such as x-ray crystallography, have been used to determine protein structures. However, these methods are time-consuming and costly, limiting the number of structures that have been mapped. This serves as the training data for learning-based approaches to the protein folding problem.
Q: What are some potential future impacts and applications of the protein folding breakthrough?
In addition to advancements in the prediction and understanding of protein structures, this breakthrough could lead to discoveries and treatments for diseases, enable the design of new proteins with desired functions, and have applications in various industries such as agriculture, healthcare, and materials science. It may also pave the way for end-to-end learning in biological systems and biophysics simulations, providing insights into complex systems at different scales, from cells to entire organs and beyond.
Takeaways
The breakthrough in protein folding achieved by AlphaFold has significant implications for various fields, including medicine, biology, agriculture, and materials science. The ability to accurately predict protein structures opens up possibilities for understanding diseases, designing new proteins, and developing innovative solutions. While the specific details of AlphaFold 2 are yet to be disclosed, the use of attention mechanisms and transformers indicates the continued trend of applying these techniques to complex problems. Overall, this breakthrough represents a major advancement in the fields of structural biology and artificial intelligence, with the potential to revolutionize our understanding of biological systems and impact real-world applications.
Summary & Key Takeaways
-
DeepMind's AlphaFold 2 has achieved a score of 87, surpassing the closest competition by 26 points and significantly improving upon its previous iteration's score of 58.
-
Protein folding, the process of going from an amino acid sequence to a 3D structure, is a crucial aspect of understanding protein function and the underlying causes of diseases.
-
AlphaFold 2's computational methods could lead to the rapid determination of protein structures, enabling discoveries of unknown gene functions, disease treatments, and advancements in materials and biophysics.
Share This Summary 📚
Explore More Summaries from Lex Fridman 📚
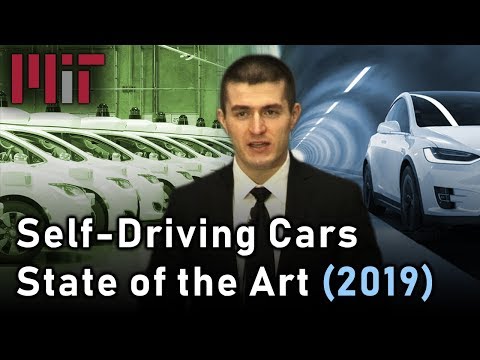
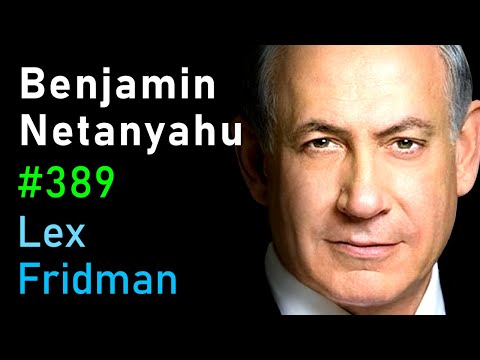

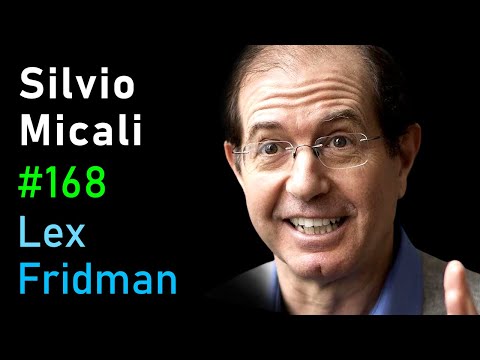
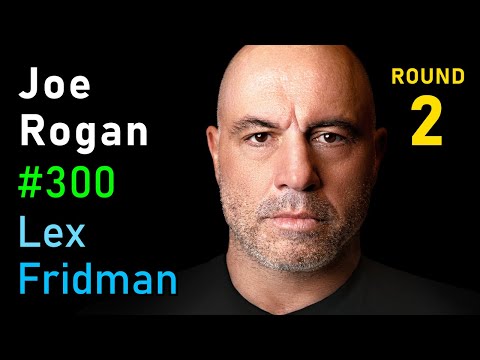
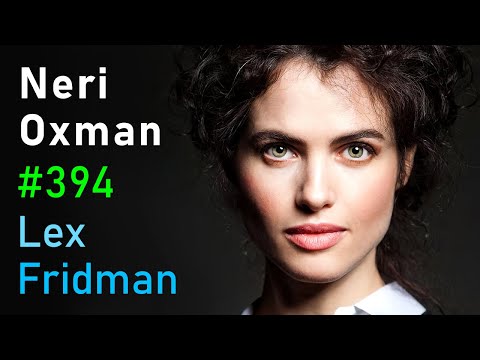