An AI For Image Manipulation Detection | Two Minute Papers #261 | Summary and Q&A
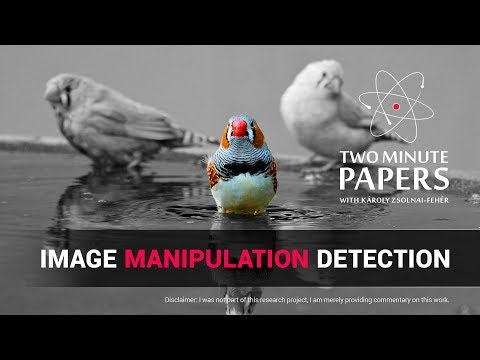
TL;DR
A learning-based algorithm can detect tampered images by analyzing color data, noise information, and their relationship, even in the presence of compression artifacts.
Key Insights
- 🕵️ Facial reenactment videos can be manipulated easily, but this algorithm focuses on detecting tampering in general images.
- ❓ The algorithm can identify image splicing, object copying, and object removal with image inpainting techniques.
- 😒 It uses a two-stream convolutional neural network to analyze color data, noise information, and their correlation for accurate detection.
- ❓ The algorithm can distinguish between compression artifacts and tampered regions, avoiding false positives.
- 🪜 Recompressing an image to add more artifacts cannot deceive the algorithm.
- 🦻 The algorithm has the potential to be repurposed for aiding in the creation of better forgeries, but its primary aim is to identify tampered images.
- 💁 It is crucial to inform the public about the existence of such tools for image manipulation detection.
Transcript
Dear Fellow Scholars, this is Two Minute Papers with Károly Zsolnai-Fehér. As facial reenactment videos are improving at a rapid pace, it is getting easier and easier to create video impersonations of other people by transferring our gestures onto their faces. We have recently discussed a technique that is able to localize the modified regions with... Read More
Questions & Answers
Q: How does the algorithm detect tampered images in general?
The algorithm uses a two-stream convolutional neural network to analyze color data and noise information in an image. It looks for unnatural contrast changes along edges and silhouettes and examines the relationship between color data and noise to identify tampering.
Q: Can the algorithm differentiate between compression artifacts and tampered regions?
Yes, the algorithm can distinguish between compression artifacts and tampered regions. It does not mistake compression artifacts for tampered areas, ensuring accurate detection of image manipulation.
Q: Can a smart attacker fool the algorithm by recompressing an image?
No, recompressing an image to add more artifacts cannot trick the algorithm. It is designed to identify anomalies and can detect tampering, even in the presence of additional compression artifacts.
Q: Can the algorithm be repurposed to aid in creating better forgeries?
While it is theoretically possible to modify the algorithm to help train a neural network for producing better forgeries, the primary purpose of the algorithm is to detect tampered images and raise awareness about the existence of such tools.
Summary & Key Takeaways
-
Facial reenactment videos can be easily manipulated, but a new algorithm can also detect tampering in general images.
-
The algorithm can identify image splicing, object copying, and object removal with image inpainting.
-
It utilizes a two-stream convolutional neural network to analyze color data, noise information, and their correlation to identify anomalies.
Share This Summary 📚
Explore More Summaries from Two Minute Papers 📚
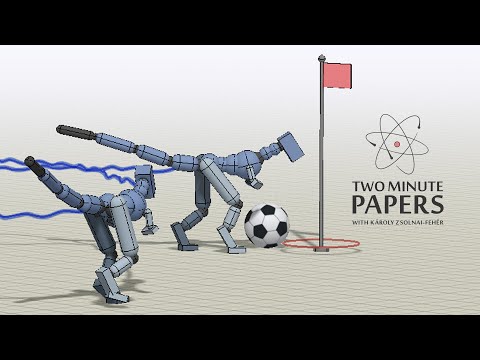
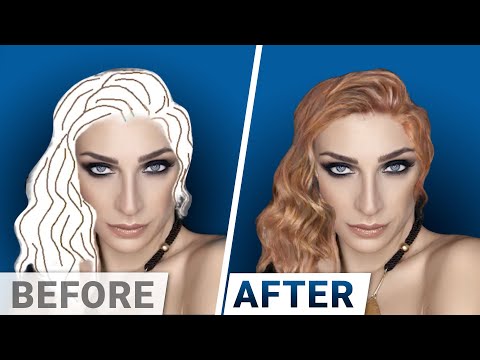
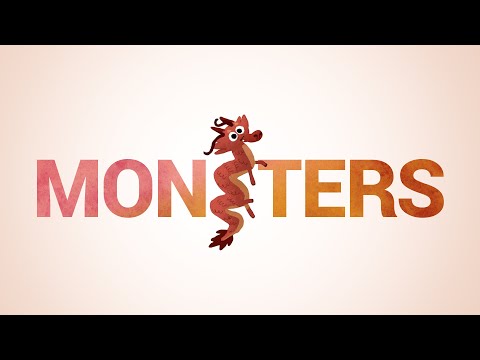
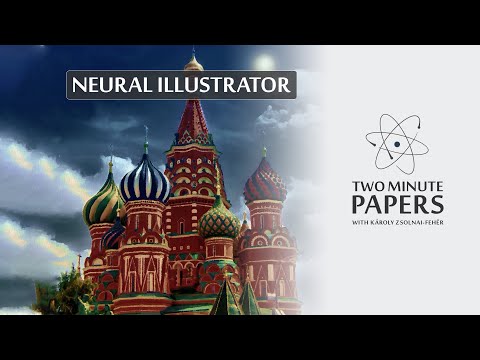
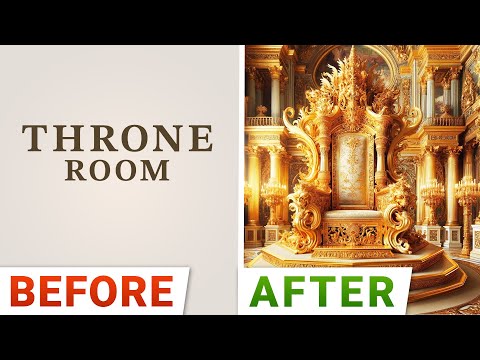
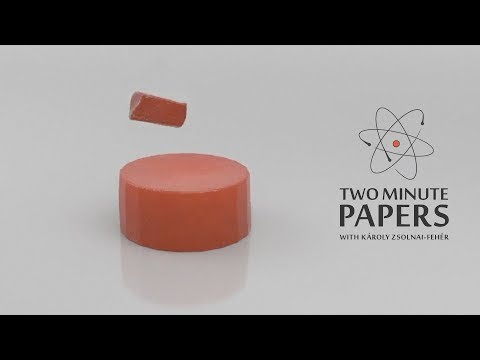